Introduction to Data Annotation and Labeling
Dive into how data annotation and labeling in cybersecurity fills key industry gaps and benefit AI.
Vaibhavi Kadam
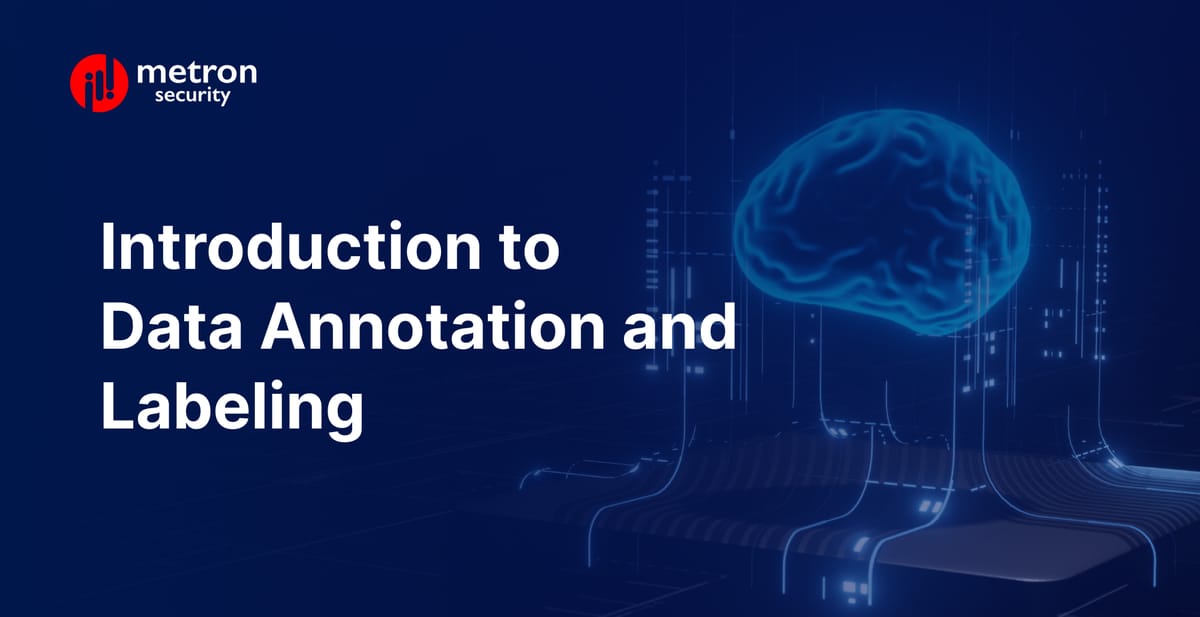
Imagine your SOC team gets alerts from both your SIEM and EDR, each one flagging the same suspicious IP address, but in completely different languages. One refers to it as “source_address”, while the other labels it as “src_ip”.
Human operators can likely make the identification, albeit at great manual cost, but what about AI? Will it be able to understand these similar warnings and cut through the noise?
Now, let’s imagine a scenario where 70% of your analysts’ time is evaporating as they manually connect dots, cross-referencing formats, decoding jargon, and chasing false leads. Sounds familiar? This is where data annotation and labeling step in as the unsung heroes bridging the gap between raw data and actionable intelligence.
In this post and the upcoming series, we’ll dive into how data annotation and labeling in cybersecurity differ from general annotation work, the key industry gaps that need attention, and how we are equipped to support you in solving these challenges.
What is Data Annotation and Labeling?
While the terms “data annotation” and “data labeling” are often used interchangeably, there is a subtle difference.
- Cybersecurity Data Labeling is about assigning predefined tags or categories to data based on specific conditions (e.g., attack type, severity, or risk). It is typically more straightforward and can be based on known patterns or behaviors.
- Cybersecurity Data Annotation can involve adding detailed metadata or contextual information to the data, often enhancing it with descriptive comments, additional observations, or even background information. You can include a more in-depth understanding of how, why, and when an event occurred.
Simply put, Annotation adds context to raw data, transforming it into a language AI can understand, while Labeling categorizes that language into clear, actionable instructions.
Why It Should Matter to SOC Teams: Without schema alignment, AI sees Splunk logs, CrowdStrike alerts, and Azure events as unrelated datasets.
Data annotation can bridge this gap and enable cross-platform correlation (e.g., linking a suspicious login in Okta to a lateral movement attempt in Palo Alto logs) along with scalability and reduced costs with automated processes.
Data Labeling vs. Data Annotation in Action: The Key Difference
In the era of modern threat detection, data labeling and annotation are the twin engines that turn cryptic alerts into actionable intel. Here’s how you can differentiate between the two and bridge the gap between noisy logs and AI-ready insights:
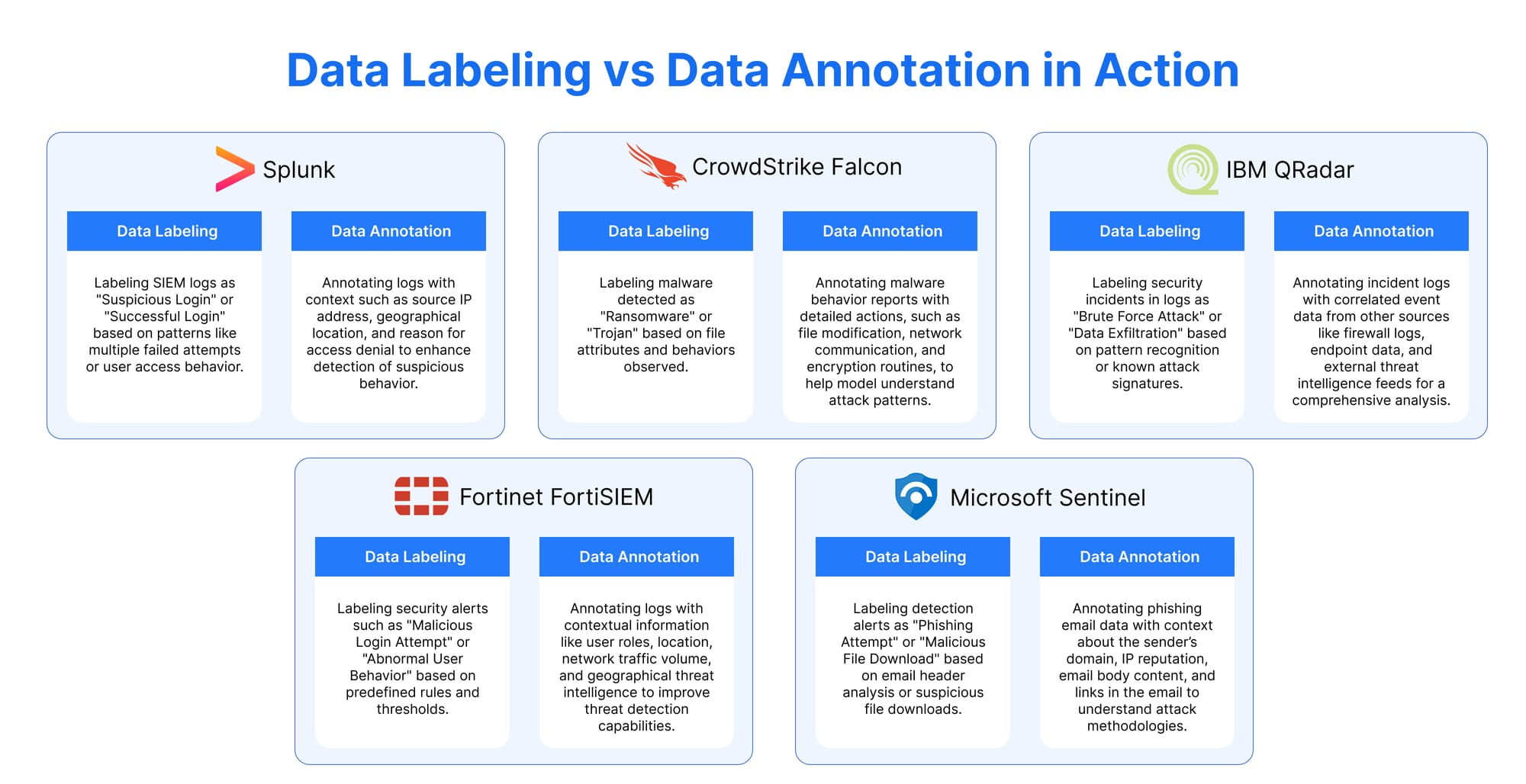
Why Data Annotation is Non-Negotiable: Key Benefits
Let’s face it, SOC teams are drowning in alerts. Multiple mid-sized companies face over 10,000+ security events daily, and most are false positives. But with annotated data, the AI model can cut through this noise. Here are a couple of key benefits of annotating your data.
Key Benefits:
- Reduced Alert Fatigue: Cortex XDR by Palo Alto Networks drastically reduced alert fatigue through intelligent grouping and deduplication, resulting in a 98% reduction in alerts. You can do it too.
- Faster Breach Resolution: With accurately labeled datasets, AI can detect and resolve breaches faster, minimizing damage and downtime.
- Assistance with GDPR & Compliance: Annotated audit trails prove due diligence; this will help organizations meet regulations like GDPR and avoid hefty fines.
- Improved Data Privacy: Secure annotation practices, like encryption and access controls, ensure sensitive data stays protected throughout the process.
The ROI of Data Annotation: Beyond Breach Prevention
Investing in annotation isn’t just about stopping threats, it’s about giving your SOC a strategic edge in a battle. According to Grand View Research, the data annotation market is expected to hit $8.22 billion by 2028, with services expanding at a 26.6% annual rate and reaching $5.3 billion by 2030. Moreover, even the global data annotation tools market, which was worth $1.02 billion in 2023, is projected to grow at 26.3% CAGR through 2030. This is the time when AI-powered solutions are becoming more essential than ever.
We are already seeing the impact:
- Microsoft Azure Sentinel uses annotated network traffic to analyze billions of signals and can detect advanced threats like nation-state attacks in real time.
- CrowdStrike Falcon annotates malware behavior reports with detailed actions like file modifications and network communication patterns, helping AI to trace attack methodologies back to groups like FIN7 and Lazarus.
It is very evident that as AI adoption accelerates, high-quality data annotation will become a key to more secure decision-making.
Conclusion:
Let’s be real: SOC teams don’t necessarily need more alerts, they need better answers. At Metron, we specialize in this behind-the-scenes work which makes AI function by aligning schemas, annotating context, and labeling threats with precision. Our team is equipped with in-depth knowledge of cybersecurity data models with battle-tested workflows to deliver annotations that turn fragmented logs into unified threat narratives.
We’re here to help you decode your first log and guide enterprises with annotation and labeling at scale. Why? Because, whether you’re defending a cloud app or an infrastructure network, AI shouldn’t be a black box: it should speak your language.
So are you ready to empower your SOC with AI that cuts through the noise? Let’s turn your data into a strategic asset. Connect with us at connect@metronlabs.com.